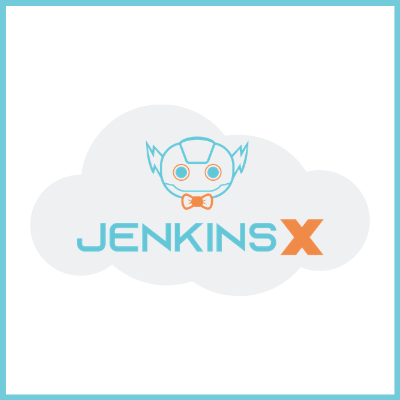
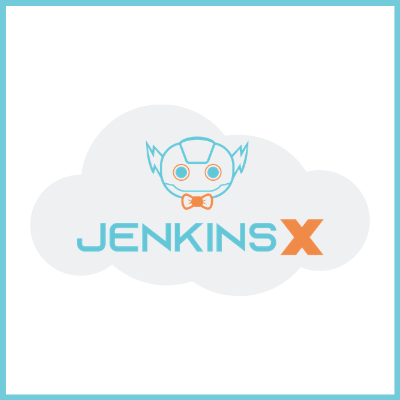
Heyyo!
My name is Steven Terrana. It’s great to be here! I’m currently a DevSecOps & Platforms Engineer at Booz Allen Hamilton.
My day to day largely consists of working with teams to implement large-scale CI/CD pipelines using Jenkins, implementing DevSecOps principles, and adopting all the buzzwords :).
Through experiencing all of the pains associated with the “large-scale” pipeline development, I developed the Jenkins Templating Engine: a Jenkins plugin that allows users to stop copying and pasting Jenkinsfiles by creating tool-agnostic, pipeline templates that can be shared across teams enabling organizational governance while optimizing for developer autonomy. If that sounds cool, you can check out the Jenkins Online Meetup.
You can probably find me somewhere in the Jenkins community. I help drive the Pipeline Authoring SIG and contribute to community plugins and pipeline documentation where I can.
I’m excited to be a part of an organization in CDF that’s helping to establish best practices, propel the adoption of continuous delivery tooling, and facilitate interoperability across emerging technologies to streamline software delivery.
Oh, yeah, and I have two cats and a turtle. Meet James Bond, GG, and Sheldon:
Follow me on Twitter @steven_terrana
Originally posted on the Harness.io blog by Tiffany Jachja (@tiffanyjachja)
In an organization where developers are continuously pushing code to production, managing risks can be difficult. In Measuring and Managing Information Risk: A FAIR Approach, Jack Freund and Jack Jones describe governance as a cost-effective approach to “govern the organization’s risk landscape.” You want to ensure your organization actively understands and manages risk, especially in heavily regulated industries expected to comply with governing authorities and standards, see compliance, or a blog post on measuring compliance.
Governance, risk management, and compliance (GRC) is an umbrella term covering an organization’s approach across these three practices: governance, risk management, and compliance. Freund and Jones describe risk and compliance.
“This [the risk] objective is all about making better-informed risk decisions, which boils down to three things: (1) identifying ‘risks,’ (2) effectively rating and prioritizing ‘risks,’ and (3) making decisions about how to mitigate ‘risks’ that are significant enough to warrant mitigation.”
“Of the three objectives, compliance management is the simplest—at least on the surface. On the surface, compliance is simply a matter of identifying the relevant expectations (e.g., requirements defined by Basel, Payment Card Industry (PCI), SOX, etc.), documenting and reporting on how the organization is (or is not) complying with those expectations, and tracking and reporting on activities to close any gaps.”
So if GRC is about aligning an organization to managing risks, what role do developers play?
We discussed in the previous blog posts the importance of taking a systematic approach to developing software delivery processes. We shared practices like Value Stream Mapping, to give organizations the tools to better understand their value streams and to accelerate their DevOps journey. These DevOps practices indicate that every software delivery stakeholder is responsible for the value they deliver. But on the flip side, they also indicate that stakeholders are responsible for any risks that they create or introduce.
The DevOps Automated Governance Reference Architecture found here, shares how to further adopt a systems approach to delivery.
By looking at each stage in your delivery pipeline, you can define the inputs, outputs, actors, actions, risks, and control points related to that stage.
The essential part of governance is that developers are aware of the risks at each stage. The reference architecture paper shares some of the common risks associated with code commits, such as unapproved changes and PII or credentials in source code. Likewise, for deploying to production, you can have risks such as low-quality code in production, lack of quality gates, and unexpected system behaviors in production.
These risks help define areas of control points that help manage that risk. If you face the risk of unapproved changes, introduce a change approval process. Likewise, you can control risks through secrets management, application quality analysis, quality gate evaluation, and enforced deployment strategies.
Everyone involved in the process from code commits to production is responsible for mitigating risks.
Now let’s discuss the components of a governance process for a cloud environment. The DevOps Automated Governance Reference Architecture, found here, shares an approach to navigating your automated governance journey. Many of these concepts to be discussed here are explained in detail in that reference paper.
Notes are metadata definitions. Occurrences are generated for each artifact or resource that needs this note. As an example, a note could provide details of a specific vulnerability, such as the impacts, names, and status. I would generate an occurrence for every container image with that security vulnerability. Similarly, I could have a note that defines a specific application deployment, as I promote the deployment across different environments, I would generate an occurrence. There is a one to many relationships between notes and occurrences.
An attestation is a particular type of note that represents a verification that you’ve satisfied in your governance process. Attestations are tied to attestors, which hold the authority to verify a control point within your governance process. For example, determining you’ve passed a code review or a unit test is an attestation. Each attestation represents a control point within your governance process.
A binary authorization policy uses a list of attestors to represent your governance as code. A binary auth policy acts as a series of gates so that you can not get to the next stage of your software delivery before getting an attestation from each attestor. Therefore, it’s common practice to turn on binary authorization (BinAuthz) in your Kubernetes environment to ensure you are governing changes and deployments. You’ll have an Admission Controller in your Google Kubernetes Engine(GKE) that does the checks for attestation when you go interact with your environment. Here’s more information on how BinAuthz works for GKE.
If you’d like to learn more about designing control points for your governance process, Captial One also shared their pipeline design through a concept called “16 Gates” in a blog post called “Focusing on the DevOps Pipeline.”
Governance processes require automation to accelerate software delivery; otherwise, it can harm your velocity and time to market. A popular topic to emerge in the past year is automated pipeline governance, which gives enterprises the ability to attest to the integrity of assets in a delivery pipeline. Pipeline governance goes beyond traditional CICD, where developers simply automate delivery without truly mitigating risk. Continuous Integration and Continuous Delivery platforms can help heavily regulated industries manage their governance processes when developers and operations understand the organization’s risks.
Originally posted on the Harness.io blog by Tiffany Jachja (@tiffanyjachja)
Value-stream mapping (VSM) is a lean manufacturing technique popularized in the 90s after its successful applications in the manufacturing industry with companies like Toyota. Since then, DevOps practitioners have shared these processes as it applies to software development. DevOps literature, such as the DevOps Handbook, suggests value stream maps inform the most critical areas of application for DevOps practices and technology. A VSM is also known as a material and information flow map. Using this map, you can identify areas of improvement and map your current state to your future state. If you’d like an in-depth look at VSM, I recommend “Value Stream Mapping: How to Visualize Work and Align Leadership for Organizational Transformation” written by Karen Martin and Mike Osterling. This blog post summarizes the key concepts of VSM and shares how you can use VSM within your IT organization.
In a VSM, three types of flow deliver a product/service to a consumer. The flow of information, the flow of materials, and the flow of time. The flow of information goes from right to left in a VSM. And in contrast, the flow of information and time goes from left to right in a VSM. The illustration below shows the major components of a VSM.
Within the information section, the factory symbol denotes the customer, supplier, and any other entities. All VSMs have a customer and supplier specified. A truck indicates the frequency of delivery. Within the materials section, the process boxes host additional space to include information about the resources needed to complete a particular process. It can be advantageous to also track inventory between processes. This is often denoted by a triangle. We also have the arrows which can denote nonlinear/sequential relationships between processes. Lastly, a VSM has a flow of time to showcase lead times in the flow of materials and information.
These are the major components of a VSM. There may be additional VSM components and symbols that are helpful for more complex value stream exercises. This resource explains those VSM components in more detail.
Example Value Stream Map (edited from a Lucid Chart Template found here).
Here are the steps to creating a value stream map:
Now that we have a VSM let’s discuss how to analyze it. If you are not adding value to your end customer, you are adding to the cost of production. Value stream management practices encourages organizations to focus on the flow of value.
Organizations will look at a Value Stream Map to consider the performance of entire systems. Starting with a single stream of value can help ease other parts of your organization into adopting new ideas and practices.
Flow refers to the flow of work in your value stream. Work can come from features requests, requirements, defects, and or support tickets. The goal is to ensure that the value stream is always moving forward. Some things that can affect flow include:
A book titled Team Topologies authored by Matthew Skelton and Manuel Pais share a few more obstacles to flow. https://teamtopologies.com/
Here is a guide to improving the flow of work within a value stream. It involves creating a dedicated transformation team that’s held “accountable for achieving a clearly defined, measurable, system-level result (e.g., reduce the deployment lead time from “code committed into version control to successfully running in production” by 50%).” This dedicated team should have the resources and freedom to utilize the different DevOps practices to achieve the results.
There are also eight types of waste defined through the lean manufacturing movement. The diagram below describes these types of waste.
The 8 Types of Waste. (Image source: https://theleanway.net/The-8-Wastes-of-Lean)
If we’d like to minimize in our software delivery processes we need to consider how to manage these findings.
Here are some more ways to look at a Value Stream Maps.
A well-executed VSM workshop gives those in the room the opportunity to champion change. Maybe you’re using an outdated tool for a certain process. A VSM workshop invites critical stakeholders to come and challenges areas of your value stream.
Every organization has a process for delivering their product or service to their customers. Value-stream mapping allows you to optimize your flow of materials and information by lowering costs and improving value adds. This blog posts shares some tips for navigating your DevOps journey through Value stream management techniques such as Value Stream Mapping.
Hello folks,
My name is Alex and I am excited to be a part of the Continuous Delivery Foundation community. I believe that the work we do here will help the world deliver faster through interoperability of technology and foundational component governance.
I am an open source contributor to many projects and also advocate for the work of the Cloud Native Computing Foundation. I hold it a great privilege to help give a platform for those without a voice and act as a force multiplier to create opportunities for others.
I have worked for the past thirteen years at Microsoft, BSkyB, Blinkbox, Beamery and more. Working as both an individual contributor and engineering leader.
Now I am an engineering director at American Express.
I work on large scale serverless runtime observability, proliferating DevOps practices and hybrid-cloud cluster development on hyper-converged infrastructure.
It is my hope that I can bring real end-user community desires and feedback to the governance body of the CDF to help us understand and shape the activities that we invest time in. Equally I am looking forward to working as an ambassador to help the community gain tangible benefits of a collaborative vendor-neutral continuous delivery ecosystem.
– Alex
Near my home in the beautiful south of England.
Hi All,
I am Hector Calderon, an engineer, architect, or insert open source loving nerd adjective here. I have worked with companies of all sizes from small to enterprise. With a vendor/cloud agnostic mentality, I have had the chance to work with many open source projects, including all of the graduate CDF projects. When I am not trying to automate myself out of a position I am out exploring the world with my dog, Piper.
My delivery journey started a few years ago when I was assigned to optimize RPM libraries for modernizing mainframes.. Yes, MAINFRAMES. It’s actually a lot cooler than it sounds and I had the opportunity to work with some of the best engineers I know to this day.
Over the years and various companies, I noticed the most important requirement for delivering success is largely based upon the mentality of the team and company (below is a meme we put on the back of our CI team shirts). Note, huge fan of Napoleon Hill’s QQMA formula – quality, quantity, and mental attitude.
At the end of the day everybody wants a high-quality product at a fast speed with least amount of overhead.
I have not been much of a contributor in the past, but now I am looking to give back to the community that has taught me so much. My objective as Ambassador is to make delivery easier for everyone. Feel free to reach out.
Stay safe and stay tuned for my next post.